Efficient Sensor Placement from Regression with Sparse Gaussian Processes in Continuous and Discrete Spaces
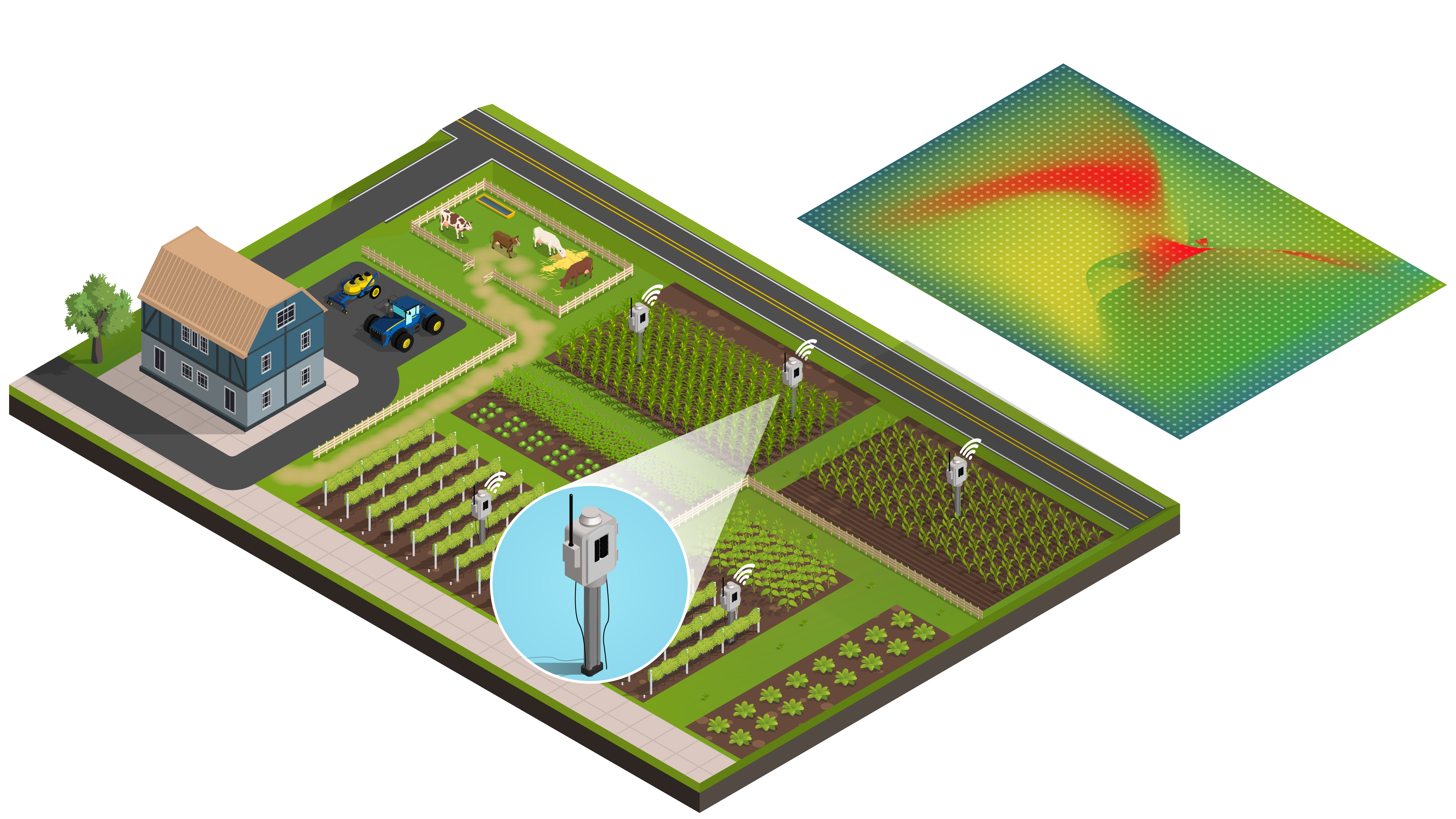
Abstract
The sensor placement problem is a common problem that arises when monitoring correlated phenomena, such as temperature, precipitation, and salinity. Existing approaches to this problem typically formulate it as the maximization of information metrics, such as mutual information~(MI), and use optimization methods such as greedy algorithms in discrete domains, and derivative-free optimization methods such as genetic algorithms in continuous domains. However, computing MI for sensor placement requires discretizing the environment, and its computation cost depends on the size of the discretized environment. These limitations restrict these approaches from scaling to large problems.